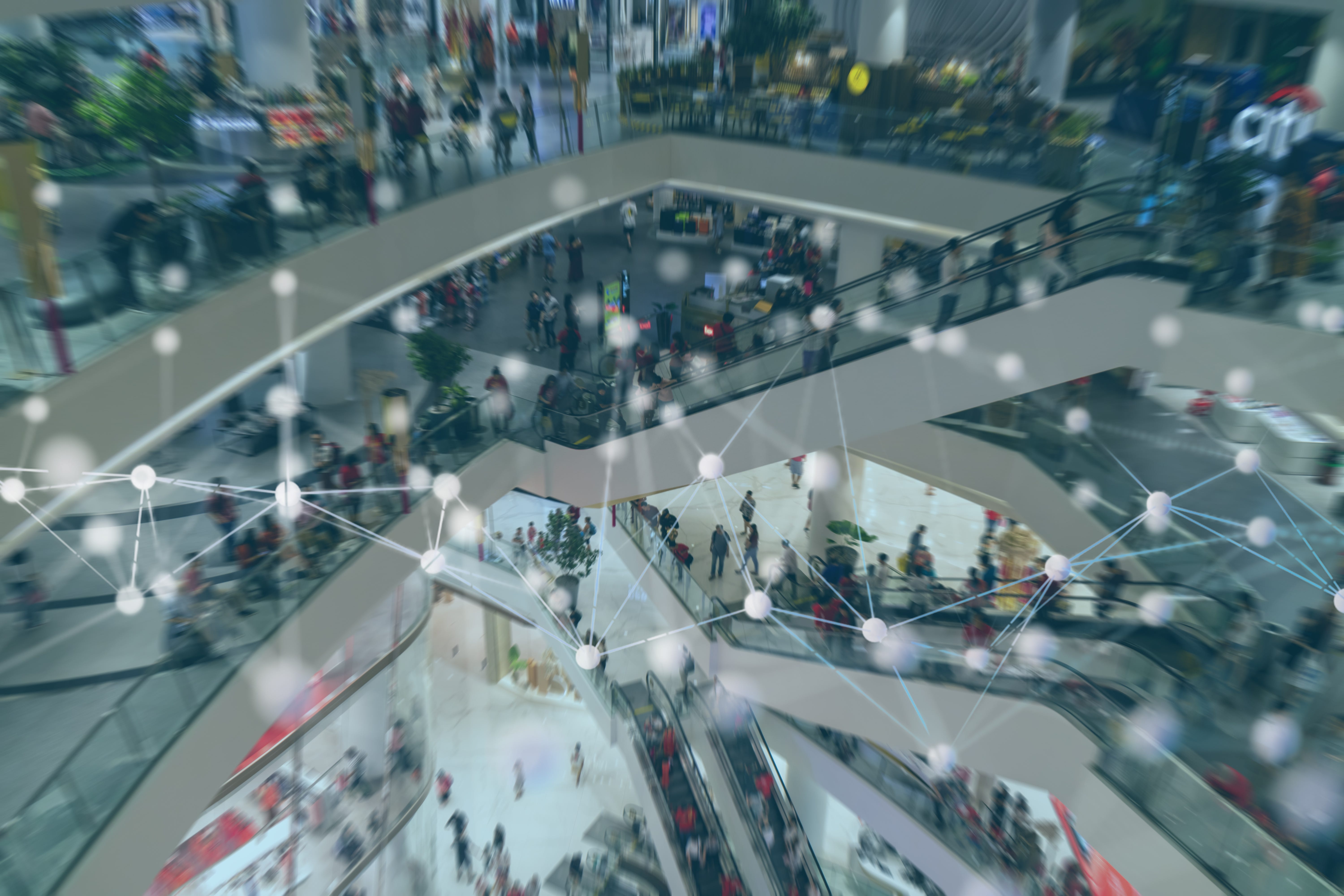
Abstract
In this article we would look at a very simple demonstration of how we can use the linear regression model to predict the estimated revenue based on the number of texts sent out in Adobe Analytics and would compare the calculations using sklearn.
(VISHNU — I need an opening for this blog. What is the purpose to the customer or the market trend that makes this step-by-step guide useful? Less than 150 words please. Here is a quick research on linear regression models to help you. Please tie in the customer value and what Adobe Analytics Workspace Analytics offers.
“Simple Implementation: Linear Regression is a very simple algorithm that can be implemented very easily to give satisfactory results. Furthermore, these models can be trained easily and efficiently even on systems with relatively low computational power when compared to other complex algorithms. Linear regression has a considerably lower time complexity when compared to some of the other machine learning algorithms. The mathematical equations of Linear regression are also fairly easy to understand and interpret. Hence Linear regression is very easy to master.
Performance on linearly separable datasets: Linear regression fits linearly separable datasets almost perfectly and is often used to find the nature of the relationship between variables.
Overfitting can be reduced by regularization: Overfitting is a situation that arises when a machine learning model fits a dataset very closely and hence captures the noisy data as well. This negatively impacts the performance of the model and reduces its accuracy on the test set. Regularization is a technique that can be easily implemented and is capable of effectively reducing the complexity of a function so as to reduce the risk of overfitting.”
Read Full Blog
Q&A
Please use this thread to ask the related questions.
Kautuk Sahni